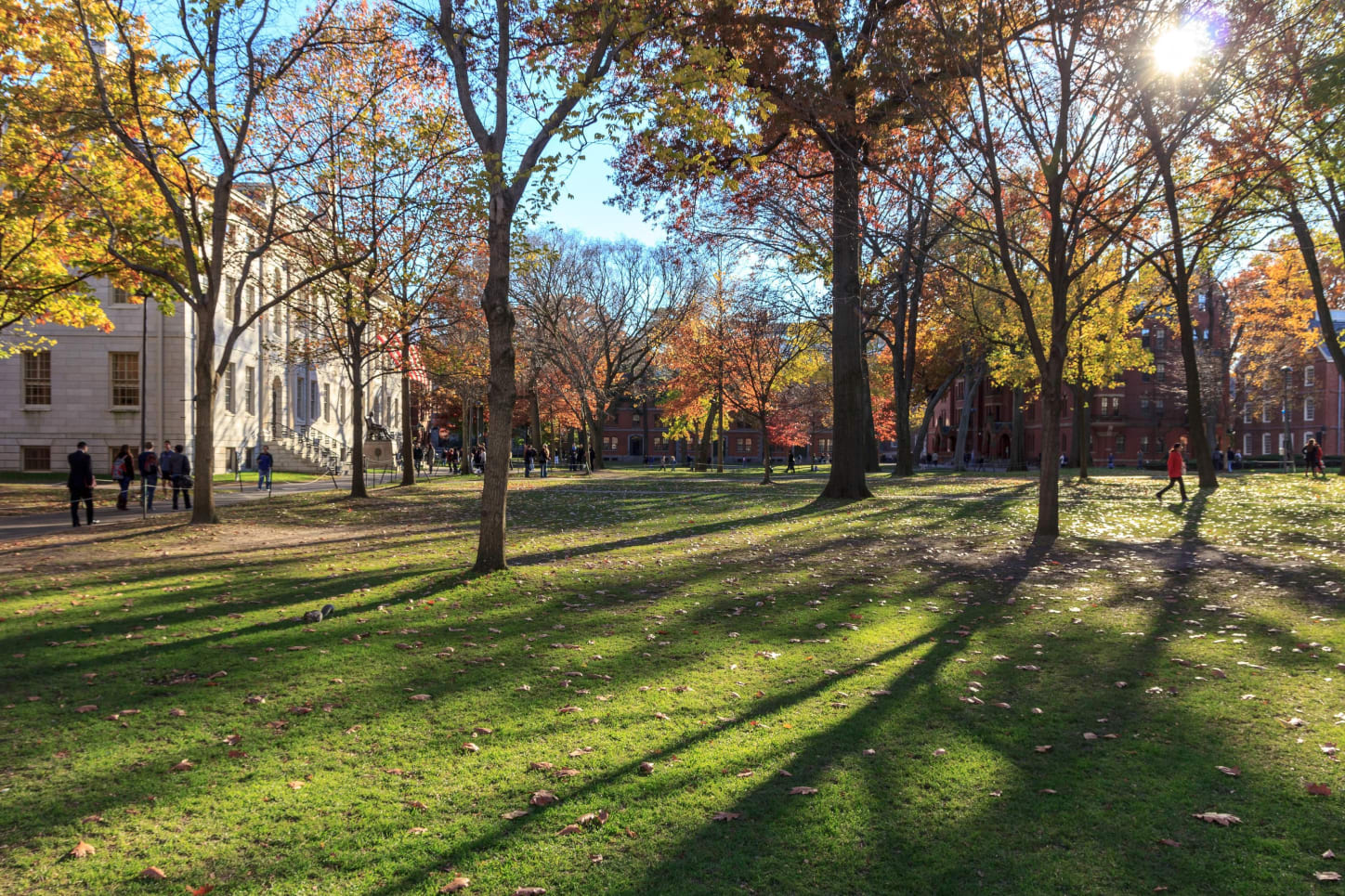
Master of Science in Computer Engineering
Stephenville, USA
DURATION
33 up to 36 Hours
LANGUAGES
English
PACE
Full time
APPLICATION DEADLINE
09 Jan 2025
EARLIEST START DATE
Mar 2025
TUITION FEES
USD 36,305
STUDY FORMAT
On-Campus
Introduction
Important Note Regarding International Students:
International students are required to be enrolled full-time (9 hours is full-time for grad) with 6 hours face-to-face and 3 hours online. INTL students can pursue Computer Engineering on the Stephenville campus, (Fall or Spring) and they can pursue the MBA at the Ft. Worth campus with a Fall-only start. International students can study online only if they remain outside the US. If they want to come to the US they have to study in person.
One other caveat..... is if an international student has an H visa, they can study online programs in the US but most of our students are on an F visa which requires them to study f2f at a campus. H visa holders are here to work and being able to go to school is an added benefit. F visa holders are here to study only and are not allowed to work.
Empower Your Future in Engineering Innovation
Advance your career and stay at the forefront of technology with the M.S. in Computer Engineering from Tarleton State University’s Mayfield College of Engineering. Whether you choose to study online or on-campus at our cutting-edge Stephenville facility, you’ll be immersed in a rigorous, hands-on program designed to propel you toward success in today’s rapidly evolving technology landscape.
Why Choose Tarleton’s M.S. in Computer Engineering?
Tarleton’s M.S. in Computer Engineering stands out by offering unmatched real-world applications of computing and engineering principles. We leverage industry partnerships and groundbreaking research to deliver a program that goes beyond theory. Our faculty collaborates directly with leading companies, ensuring students engage with cutting-edge problems and emerging technologies from day one. Unlike similar programs, you’ll benefit from small class sizes, personalized mentorship, and access to state-of-the-art research labs – often reserved for graduate students at larger institutions.
Program Features
- Flexible Learning Options for International Students: Enroll full-time (9 hours) with 6 hours face-to-face at the Stephenville Campus, and 3 hours can be completed online.
- Hands-On Learning: Apply what you learn to real-world challenges, gaining experience with research projects and advanced tools.
- Specializations for the Future: Concentrate in areas like VLSI Circuit Design, Cybersecurity, Robotics, and Artificial Intelligence.
Unique Curriculum Specializations
Our program is renowned for its advanced focus on optimization and stochastic models, a unique combination rarely found at other institutions. You'll gain expertise in areas such as:
- Computer Architecture & Distributed Computing
- Advanced Computer Networks (with a strong focus on Cybersecurity)
- VLSI Circuit Design
- Robotics, Artificial Intelligence, and Machine Learning
Thesis or Non-Thesis Options
Tailor your educational experience to fit your career goals. The thesis option provides deep research opportunities, while the non-thesis professional track offers a practical approach designed to enhance your industry readiness.
Unmatched Marketable Skills
Our program goes beyond just technical expertise. You’ll graduate with a robust set of marketable skills, including:
- Complex Problem-Solving: Solve critical issues in fields like aerospace, medicine, robotics, and military technology.
- Technical Mastery: Learn advanced math and programming languages, including C/C++, while staying agile in the evolving landscape of technology.
- Collaborative and Communication Skills: Work effectively with teams, end-users, and stakeholders to design innovative solutions.
Real-World Research Experience
Tarleton’s M.S. in Computer Engineering offers unique research opportunities that enhance your educational journey. Collaborate with faculty members who are conducting groundbreaking work in areas like wireless communication systems, network teletraffic modeling, robotics and AI, and cybersecurity. Research isn't just a theoretical exercise here; it's a way to expand your resume, build networks, and even earn stipends as you contribute to meaningful innovations.
Distinguished Faculty
Your learning experience will be guided by a team of expert faculty with vast industry and research backgrounds. Each of our faculty members brings hands-on experience and industry connections, helping you bridge the gap between academic theory and real-world application.
State-of-the-Art Facilities
The Mayfield College of Engineering’s modern facilities include advanced teaching labs, maker spaces, and research equipment that rival the resources of larger institutions. Our recent $54 million investment in a new building underscores our commitment to providing a top-tier learning environment. Whether you’re building cutting-edge AI systems or working on next-gen wireless networks, you’ll have the tools you need to succeed.
Prepare for the Future – Enroll Today
Texas is a growing hub for engineering and computer science professionals, with Tarleton graduates at the forefront of this expansion. The U.S. Bureau of Labor Statistics projects robust growth in engineering fields, and Texas ranks among the top states for employment and wages in these industries.
Choosing Tarleton’s M.S. in Computer Engineering means choosing a future filled with opportunity, innovation, and success. Apply today to start your journey toward a career that shapes the world!
By choosing Tarleton State University’s M.S. in Computer Engineering, you’re not just earning a degree – you’re gaining the skills, mentorship, and opportunities to transform your future.
Admissions
Curriculum
CPEN 5099. Thesis Research. 1-6 Credit Hours (Lecture: 1-6 Hours, Lab: 0 Hours).
Research for Master’s thesis in Computer Engineering Prerequisites: Graduate standing.
CPEN 5341. Advanced Algorithms. 3 Credit Hours (Lecture: 3 Hours, Lab: 0 Hours).
Amortized analysis, graph, network flow, string matching, matrix and polynomial algorithms, linear programming, NP-completeness, approximation algorithms, and an introduction to parallel algorithms. Prior knowledge or experience in data structures and algorithms is recommended. Prerequisite: Approval of department head.
CPEN 5342. Parallel Computing and Algorithms. 3 Credit Hours (Lecture: 3 Hours, Lab: 0 Hours).
Taxonomy of parallel computers, shared-memory and message-passing architectures, theoretical models; patterns and strategies for designing parallel algorithms; parallel data structures; automatic parallelization of sequential programs; communication; synchronization and granularity; applications. Prior knowledge or experience in Computer Architecture is recommended.
CPEN 5343. Advanced Computer Architecture. 3 Credit Hours (Lecture: 3 Hours, Lab: 0 Hours).
The course is structured around the three primary building blocks of general-purpose computing systems: processors, memories, and networks. Topics include the limitations of scalar pipelines, superscalar execution, out-of-order execution, register renaming, memory disambiguation, branch prediction, and speculative execution; multithreaded, VLIW, and SIMD processors; non-blocking cache memories, and memory synchronization, consistency, and coherence; multi-core, shared-memory architectures. The course also covers techniques for quantitative analysis of computer systems, to understand and compare alternative design choices. Prior knowledge or experience in Computer Architecture is recommended. Prerequisite: Approval of department head.
CPEN 5348. Advanced VLSI Circuit Design. 3 Credit Hours (Lecture: 3 Hours, Lab: 0 Hours).
Analysis and design of key analog and mixed-signal IC blocks: analog switches, sampling circuits, switched-capacitor filters, ADCs, DACs, and PLLs. Low-power design techniques and machine learning applications for analog and mixed-signal ICs. Prior knowledge or experience in Electronics II and Digital Signal Processing is recommended. Prerequisite: Approval of department head.
CPEN 5351. Introduction to Convex Optimization. 3 Credit Hours (Lecture: 3 Hours, Lab: 0 Hours).
This course introduces convex optimization problems, the basics of convex analysis, algorithms for convex optimization and their complexities, and applications of convex optimization. The course also trains students to recognize convex optimization problems that arise in scientific and engineering applications and to use software tools to solve convex optimization problems. Prior knowledge or experience in Calculus III and Matrix Algebra is recommended. Prerequisite: Approval of department head.
CPEN 5355. VLSI Architectures. 3 Credit Hours (Lecture: 3 Hours, Lab: 0 Hours).
The course covers the most important methodologies for designing custom or semi-custom VLSI systems for typical signal processing and communications applications. Techniques for the inner and outer receiver, mapping of algorithms onto array structures, digital signal processing (DSP) systems, and field programmable gate arrays (FPGAs), programmable signal processors. Prior knowledge or experience in Computer Architecture is recommended. Prerequisite: Approval of department head.
CPEN 5361. Deep Neural Networks. 3 Credit Hours (Lecture: 3 Hours, Lab: 0 Hours).
Introduction to the principles and theory of neural networks, with emphasis on deep neural networks. Topics include convolutional networks, recurrent and LSTM networks, reinforcement learning, preprocessing, regularization, tuning, and optimization, as well as mathematical and programming tools. Applications to classification, image recognition, and autonomous vehicles. Prior knowledge or experience in Data Science, Machine Learning is recommended. Prerequisite: Approval of department head.
CPEN 5366. Robot Vision. 3 Credit Hours (Lecture: 3 Hours, Lab: 0 Hours).
This course aims to bridge the gap between computer vision and deep learning. It covers topics such as object detection and recognition, machine learning algorithms for computer vision, and advanced techniques for 3D computer vision. Real-world applications and projects will be implemented in the areas of autonomous vehicles and robotics. Prior knowledge or experience in Computer Vision, Python, and C/C++ programming is recommended. Prerequisite: Approval of department head.
CPEN 5377. Wireless and Mobile Communication Networks. 3 Credit Hours (Lecture: 3 Hours, Lab: 0 Hours).
Advanced architectures for wireless communication networks; advanced wireless technologies; challenges and issues in designing such networks; queueing theory and other stochastic models. Prior knowledge or experience in Computer Networks or Communication Systems Theory, Probability, one semester of programming is recommended. Prerequisite: Approval of department head.
CPEN 5378. Advanced Computer Networks. 3 Credit Hours (Lecture: 3 Hours, Lab: 0 Hours).
This course concentrates on routing and inter-networking in IP networks while addressing contemporary topics like wireless networks, security, voice and video over IP, the Internet of Things (IoT), software-defined networking, and network virtualization. Prior knowledge or experience in Computer Networks is recommended. Prerequisite: Approval of department head.
CPEN 5379. Performance of Computer and Communication Networks. 3 Credit Hours (Lecture: 3 Hours, Lab: 0 Hours).
Application of probability, Markov chains, and queueing theory to the analysis and design of computer and communication networks. Case studies in traffic shaping and multiplexing, static routing, dynamic routing, and peer-to-peer file-sharing systems. Both continuous-time and discrete-time models are explored. Prior knowledge or experience in Computer Networks or Communication Systems Theory, Probability is recommended. Prerequisite: Approval of department head. Computer Science Courses COSC 5086. Advanced Special Problems in Computer Science. 1-6 Credit Hours (Lecture: 1-6 Hours, Lab: 0 Hours). Advanced special problems in computer science. Work may be either theory or laboratory. May be repeated with the approval of the department head for additional credit.
COSC 5088. Thesis Research. 1-6 Credit Hours (Lecture: 1-6 Hours, Lab: 0 Hours).
Research for Master’s thesis in AI and Machine Learning (AIML-MS). COSC 5330. Simulation. 3 Credit Hours (Lecture: 3 Hours, Lab: 0 Hours). Introduction to simulation with emphasis on simulation methodology, random number generation, time flow mechanisms, sampling techniques, and validation and analysis of simulation models and results. Simulation languages and their applications will be investigated.
COSC 5345. Reinforcement Learning. 3 Credit Hours (Lecture: 3 Hours, Lab: 0 Hours).
This course will provide an introduction to, and comprehensive overview of, reinforcement learning (RL). Topics include Markov decision process and dynamic programming, Monte-Carlo methods, temporal difference learning, integration of planning and learning, policy gradient and actor-critic methods, deep learning, and deep RL algorithms. Students will engage in exercises and projects that involve coding in simulated RL environments. Credit will not be awarded for both COSC 4345 and 5345. Graduate students will have to complete additional assignments. Prerequisite: Advanced background in statistics and artificial intelligence.
COSC 5346. Robotics and Autonomous Systems. 3 Credit Hours (Lecture: 3 Hours, Lab: 0 Hours).
Overview of the major areas of robotics and autonomous systems. AI, machine learning, and optimization algorithms enable autonomous agents to operate in unstructured, dynamic environments, including localization and mapping, sensor fusion, computer vision, path planning, communication, and obstacle avoidance. Students will engage in exercises and projects that involve developing robotics systems with autonomous actions and evaluating their performance using computer simulations and physical robotic systems. Credit will not be awarded for both COSC 4346 and 5346. Graduate students will have to complete additional assignments. Prerequisite: Advanced background in statistics, linear algebra, and artificial intelligence.
COSC 5347. High-Performance Computing. 3 Credit Hours (Lecture: 3 Hours, Lab: 0 Hours).
This course provides an introduction to programming massively parallel processors and the architectures therein. It covers methods to harness the potential of Graphical Processing Units (GPUs) and parallel algorithms using the CUDA parallel computing platform. Algorithms from the fields of Scientific Computing, Machine Learning, and Computer Vision are introduced and explored.
COSC 5352. Optimization for Machine Learning. 3 Credit Hours (Lecture: 3 Hours, Lab: 0 Hours).
This course will explore the theory and algorithms that arise in machine learning and modern data analysis. The topics will be tailored with a particular focus on the complexity, implementation, robustness, and scalability of algorithms to large datasets. Students will engage in exercises and projects that involve programming optimization algorithms, and evaluating their performance.
COSC 5360. Artificial Intelligence. 3 Credit Hours (Lecture: 3 Hours, Lab: 0 Hours).
Introduces representations, algorithms, and architectures used to build intelligent systems. Predicate calculus, state-space representation, and search, heuristic search, knowledge-based problem-solving, symbol-based and connectionist machine learning, intelligent agents, and robotics.
COSC 5361. Deep Neural Networks. 3 Credit Hours (Lecture: 3 Hours, Lab: 0 Hours).
Introduction to the principles and theory of neural networks, with emphasis on deep neural networks. Topics include convolutional networks, recurrent and LSTM networks, reinforcement learning, preprocessing, regularization, tuning, and optimization, as well as mathematical and programming tools. Applications to classification, image recognition, and autonomous vehicles. Credit will not be awarded for both COSC 4361 and 5361. Graduate students will have to complete additional assignments. Prerequisite: Advanced background in statistics, linear algebra, and artificial intelligence.
Program Outcome
Students in our program will:
- Be able to understand logic (circuit) design.
- Be able to identify and solve complex technology problems in robotics, aerospace, business, medicine, military, and other essential areas.
- Be able to apply and adapt theoretical principles to develop new computer software and/or hardware.
- Be able to apply Computer-related math skills, e.g., linear algebra, calculus, statistics, discrete mathematics, and optimization in real-world problems.
Career Opportunities
Cutting-Edge Career Opportunities
With a Master’s in Computer Engineering from Tarleton, your career prospects are vast. Graduates find success in roles such as:
- Computer and Information Research Scientists
- Information Security Analysts
- Software Developers
- Robotics and AI Specialists
The program's alignment with industry demand ensures you’ll be equipped to take on the challenges of tomorrow’s tech landscape.